Segment Anything in High Quality (HQ-SAM): a new Foundation Model for Image Segmentation (Tutorial)
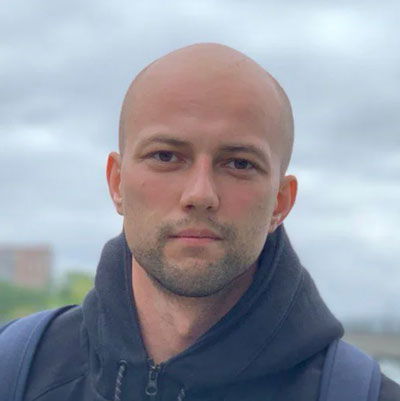
How to use new version of Segment Anything + detailed comparison with original SAM model.
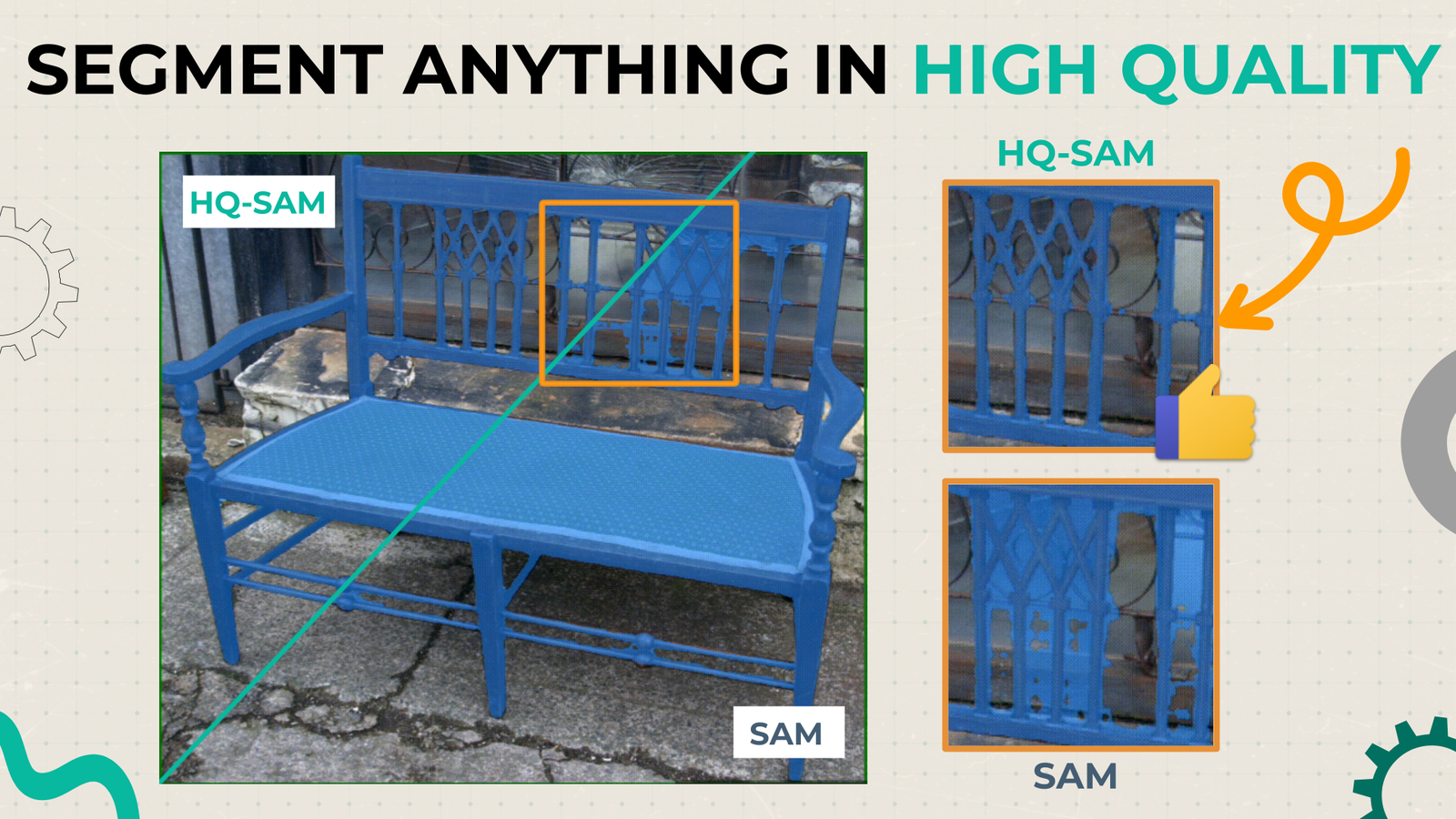
Table of Contents
Precise segmentation of diverse objects on images is fundamental for a variety of scene understanding applications, including robotic, AR/VR, image/video editing, and of course annotation of custom training datasets.
Recently introduced Segment Anything Model (SAM) can segment any object in any image using various input prompts (user feedback), including points, boxes and masks. Although it was trained on 1.1 billion masks, SAM's ability to predict masks often lacks precision, especially when it comes to objects with complex shapes and intricate structures.
The new upgraded state-of-the-art interactive segmentation model called Segment Anything in High Quality (HQ-SAM). And we are happy to announce its integration into Supervisely Ecosystem π. Now it will be easy for you to integrate this new model into custom annotation pipelines.
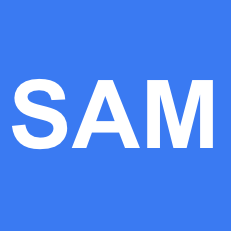
Serve Segment Anything Model
Deploy model as REST API service
Serve Segment Anything in High Quality
Run HQ-SAM and then use in labeling tool
In this tutorial, we will show you how to run and use both SAM and HQ-SAM models inside Supervisely image annotation toolbox in a few clicks. Also, we will compare these models on the hard-to-segment edge cases.
Video tutorial
Check out the video step-by-step guide on how to deploy and use models in Supervisely Computer Vision Platform. We encourage you to try both versions of Segment Anything model on your data in our free Comunity Edition.
Follow the steps:
-
Connect your computer with GPU using this 90 seconds video guide. This is a one-time procedure, if you already connected your computer, just skip this step.
-
Segment Anything (SAM) and Segment Anything in High Quality (HQ-SAM) models are integrated in the form of Supervisely Apps. Just press the "Run" button to start them on the GPU computer connected to your account.
-
Select the SmartTool in the image labeling toolbox, put the bounding box around the object of interest and correct predictions with π’ positive and π΄ negative clicks.
Go to Start β Neural Network β Interactive Segmentation.
What is Segment Anything model (SAM)?
Segment Anything model (SAM) is a foundation vision model for general image segmentation that segments a wide range of objects, parts, and visual structures in diverse scenarios, by taking a prompt consisting of points, a bounding box, or a coarse mask as input. It also can work in zero-shot segmentation scenarios when the model takes the images and predicts the masks for objects without specifying the exact class name for them. The model was introduced by Facebook Research team and was trained on billion of annotated images.
What is Segment Anything in High Quality model (HQ-SAM)?
Segment Anything in High Quality model (HQ-SAM) is an extension of the original Segment Anything model which predicts more accurate object segmentation and reuses the pre-trained model weights of SAM, while only introducing minimal additional parameters injected into SAMβs mask decoder. It was released by VIS Group at ETH ZΓΌrich. The authors compose a dataset of 44K fine-grained masks from several sources and trained the model in just about 4 hours on 8 GPUs.
HQ-SAM model demonstrated its efficiency on 9 diverse segmentation datasets across different downstream tasks, where 7 out of them are evaluated in a zero-shot transfer protocol. At the same time, the HQ-SAM model has a comparable inference speed.
HQ-SAM outperforms SAM on zero-shot coco
HQ-SAM model produces more accurate masks with the same number compared to default SAM according to the comprehensive evaluation of benchmarks.
More precise masks with the same number of clicks
Compare predictions: HQ-SAM vs SAM
HQ-SAM produces better and more accurate masks for objects with complex structure.
Here is the table with additional comparisons provided by authors. HQ-SAM is on the left, SAM is on the right.
![]() |
![]() |
![]() |
![]() |
![]() |
![]() |
HQ-SAM limitations
In the video tutorial above we found that HQ-SAM model can perform well in general but it still struggles to segment some objects precisely. Also, the model is very sensitive to the placement of the points
If the model does not work on your data as expected, check out his tutorial on how to Train custom interactive segmentation model (SmartTool) on your data in Supervisely. The customization will allow you to significantly speed up the manual annotation process on your objects.
Conclusion
In this guide, we explained how to deploy interactive segmentation models (SAM and HQ-SAM) in Supervisely Platform and easily use them inside the image annotation toolbox to automatically segment objects of interest.
Supervisely for Computer Vision
Supervisely is online and on-premise platform that helps researchers and companies to build computer vision solutions. We cover the entire development pipeline: from data labeling of images, videos and 3D to model training.
The big difference from other products is that Supervisely is built like an OS with countless Supervisely Apps β interactive web-tools running in your browser, yet powered by Python. This allows to integrate all those awesome open-source machine learning tools and neural networks, enhance them with user interface and let everyone run them with a single click.
You can order a demo or try it yourself for free on our Community Edition β no credit card needed!